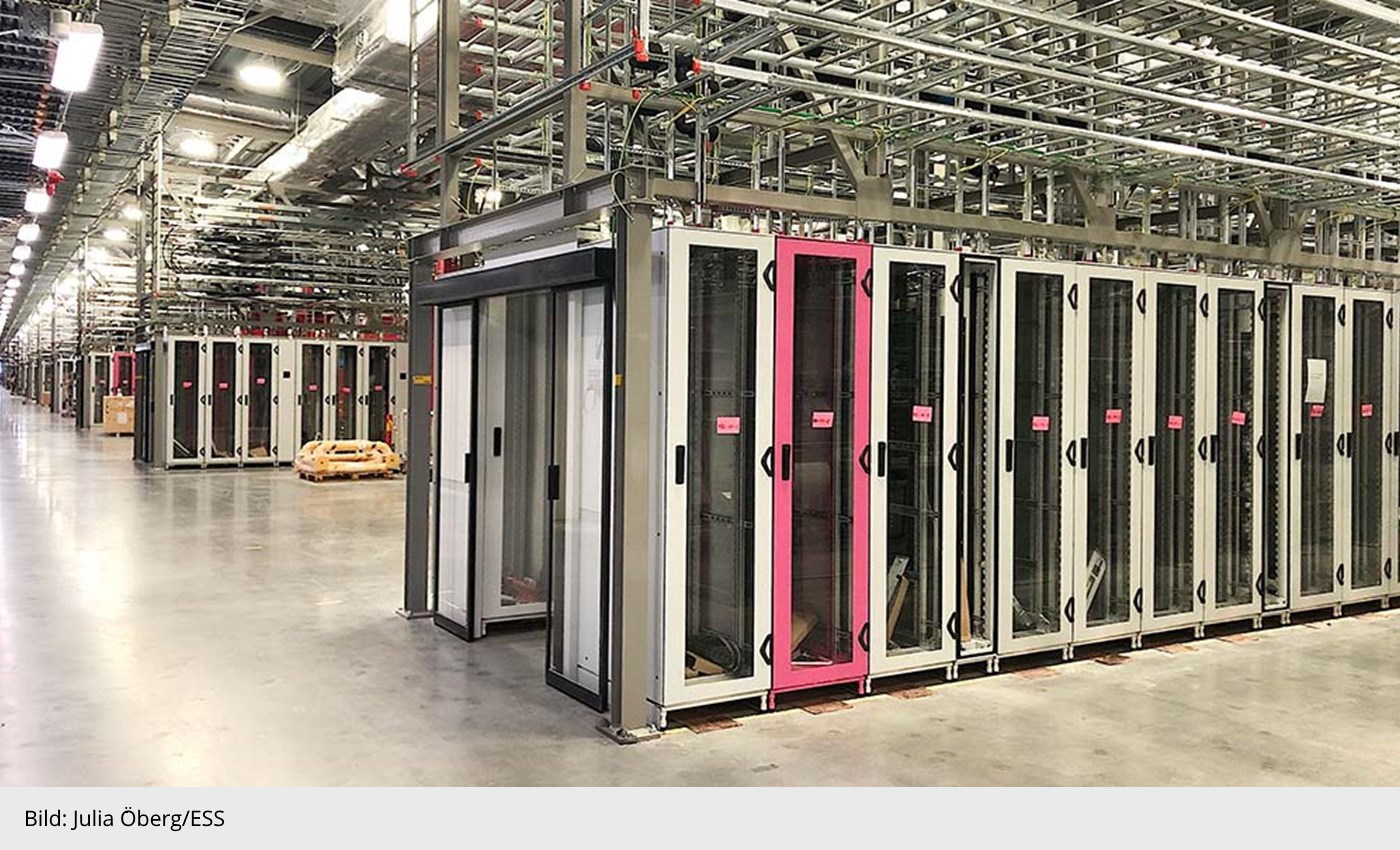
Vi har startat ett samarbete med ESS i ett nytt Vinnova-projekt där målet är att implementera algoritmer i styrsystemet för att förbättra driftsäkerheten på ESS. Denna typ av förutsägande maskininlärning är också av intresse för tillverkningsindustrin för att förhindra maskinstopp.
The ESS Integrated Controls System Division initiated a machine learning project just over a year ago with the overall goal of improving the operational reliability of the facility. DVel is now developing a machine learning algorithm whose purpose is to anticipate problems during operation before components break down or the process stops. The algorithm will be implemented in the ESS control system EPICS.
Reliability is a major challenge not only for ESS but for larger facilities in general. The difficulty for ESS and other research facilities is the enormous complexity, with over hundreds of thousands of components and millions of measurement and control points. The challenge for traditional systems in, for example, the process industry is similar. The complexity increases with more sensors, larger data volumes and a higher degree of automation. In other words, the technological development generated by the project will be of interest to a number of industries, such as the aerospace industry and healthcare.
Big Science Sweden has helped us with contacts at ESS and with several large research facilities in the world.
“The purpose of this project is to improve operational reliability and thereby provide researchers with optimal conditions for their experiments at the facility. Collaborations with industry are valuable to us because we must always ensure the quality of algorithms and integrate these with the control system on an industrial scale. ”
“We are pleased with this collaboration and see that around ESS and MAX IV, many support companies are needed to support the operation and development of the facilities. It is also exciting that DVel will be a bridge of knowledge between ESS and the manufacturing industry. ”
“We see a great need for predictive control systems throughout the process industry and hope to be able to offer effective solutions in the area.”
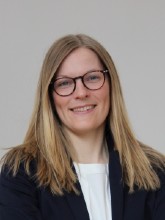
Karin Hellqvist
CSO